The success of DeepSeek with the release of their reasoning language model R1 is making the impact of recent open source development more obvious to the market.
1. Smaller models have for years now closely trailed state of the art models with a fraction of the number of parameters and fraction of compute needed. The age of exponential growth of models seems over, as we find better techniques than brute force scaling to learn more efficiently. In recent years (cf. Below) the focus of the open-source community has been to build smaller models close in accuracy to their much larger counterparts, but 100 times smaller and more economic to train/use. Biggest players on these open-source lightweight Large Language Models have been Meta (with Llama models), Google (Gemma), Ali Baba (Qwen), Mistral (Mixtral) and now DeepSeek.
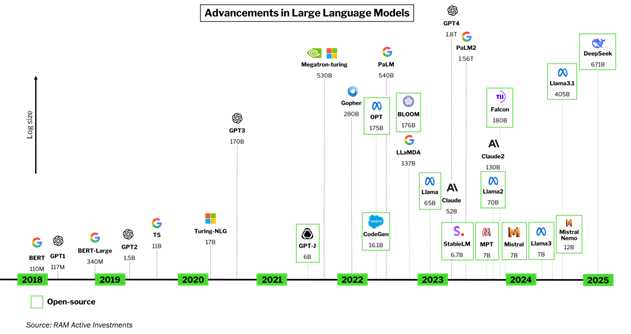
2. China vs. US: Chinese labs have been leading the AI front with US labs for years now. Chinese Large Language Models (LLMs) and derived models have topped open-source leaderboards for quarters. Not new with DeepSeek.
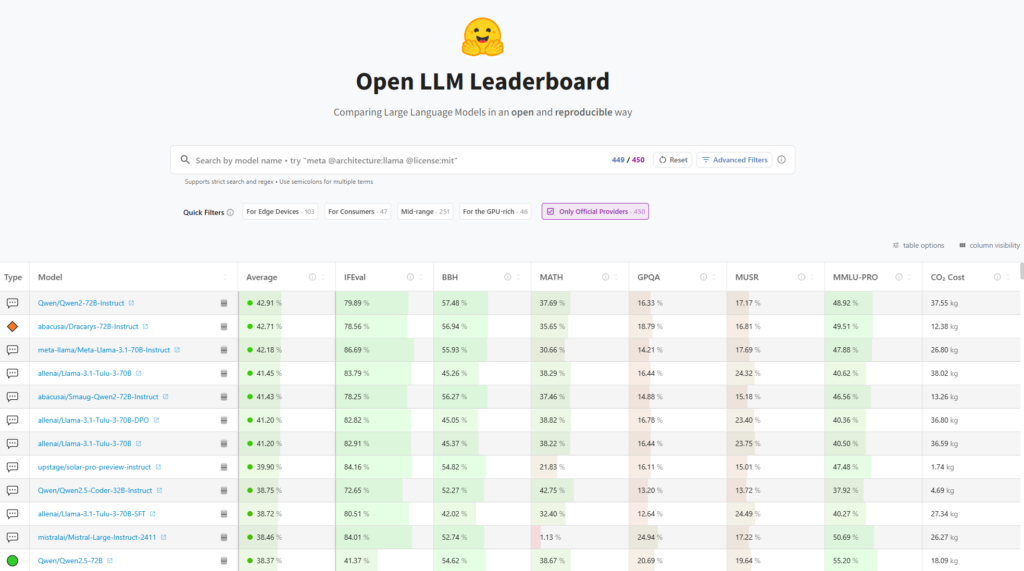
3. The most recent model developed by DeepSeek, R1, is particularly impactful as its reasoning capabilities match and sometimes are ahead of OpenAI o1 model.
Process of training DeepSeek-R1 making the headlines today:
A first base model, DeepSeek-V3_Base, was built using a Mixture-of-Experts approach, which has the advantage of being very efficient, 37bn only of 671bn parameters being activated for each token.
Pure Reinforcement Learning (learning from the quality/coherence of text outputs without a specific target/supervision) is then used to improve this base model, to make it capable of reasoning, getting DeepSeek-R1-Zero. Innovations like learning from group of outputs rather than individual outputs helps this RL be very efficient in terms of training cost.
A succession of supervised fine-tuning and reinforcement learning sequentially helps further improve/refine the model and its outputs.
The final model, DeepSeek-R1, beats OpenAI o1 at maths (AIME 224 benchmark) and matches its results at a variety of tasks. Given the efficiency of the mixture of experts model, using it costs a fraction of an o1 model (R1: $0.55 per million input tokens and $2.19 per million output tokens vs o1: $15 per million input tokens and $60 per million output tokens)
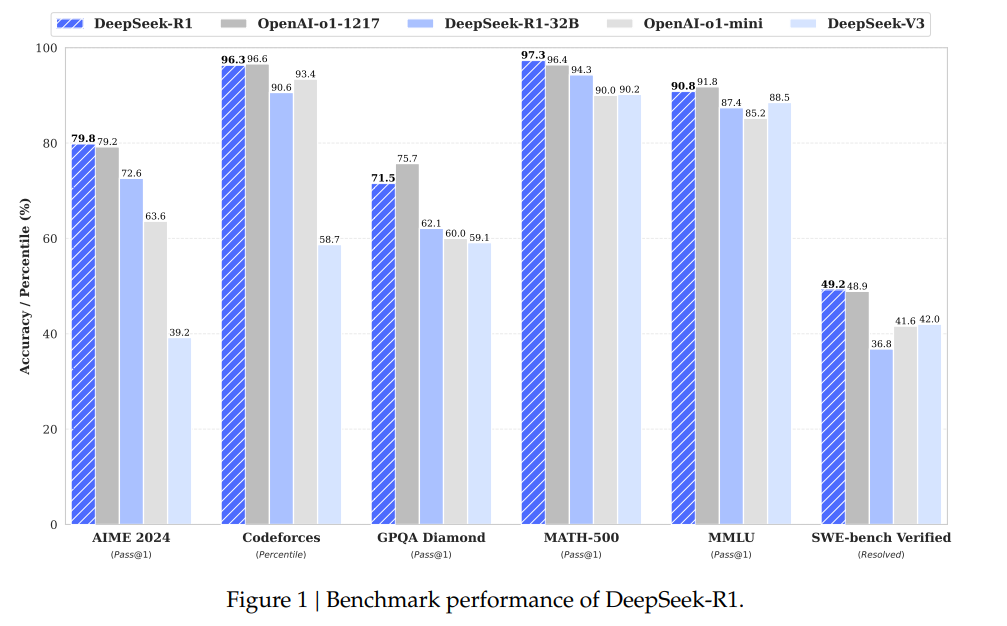
4. DeepSeek has also open sources smaller distilled models of 7bn to 70bn parameters (built with open-sources Llama and Qwen models) which are beating all models of similar sizes across reasoning, maths and coding tasks, which can be run on smaller GPU infrastructures and offer larger integration potential.
5. OpenAI dominance: the fact that DeepSeek today provides the world with a much cheaper alternative to o1 and also open-sourced very strong smaller models is a clear threat to OpenAI, which is still burning billions in employee and model training costs a year.
6. Nvidia: the (not new) development of much smaller “lightweight” models which closely trail the performance of models magnitudes larger is a welcome news for users, for the ecology, but bad news for Nvidia and infrastructure providers. The trend to more efficient models originated from open-source efforts and the expected trend of specialization of models (Mixture of Experts could be considered as part of that trend) should lead to a much more efficient use of LLM for each domain of expertise. The continuous improvement of training techniques to miminize training costs (after years of brute force of piling neural network layers) should also contribute to a slowdown in the growth of AI-related Capex.
7. In a remarkable twist, DeepSeek announced on Monday January 27th Janus-Pro-7B, an advances version of their former Janus model, which beats OpenAI DALL-E 3 at text-to-image generation, a new attack on OpenAI AI current dominance. The model is open-source and available on DeepSeek’s github page.
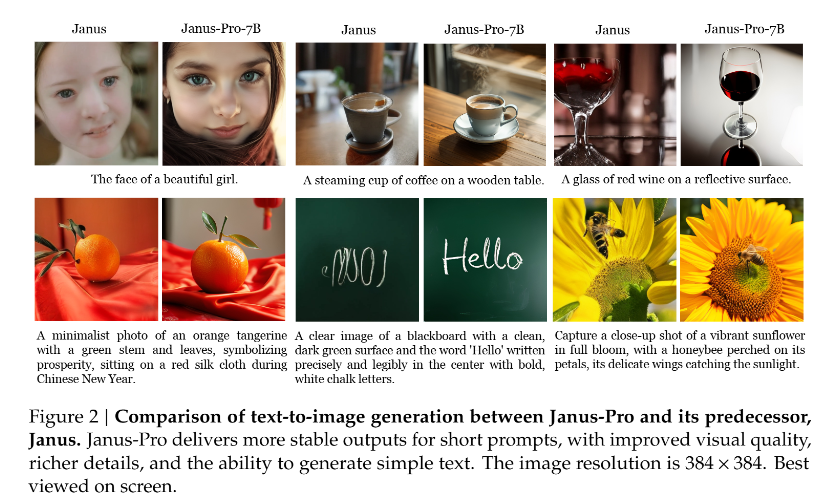
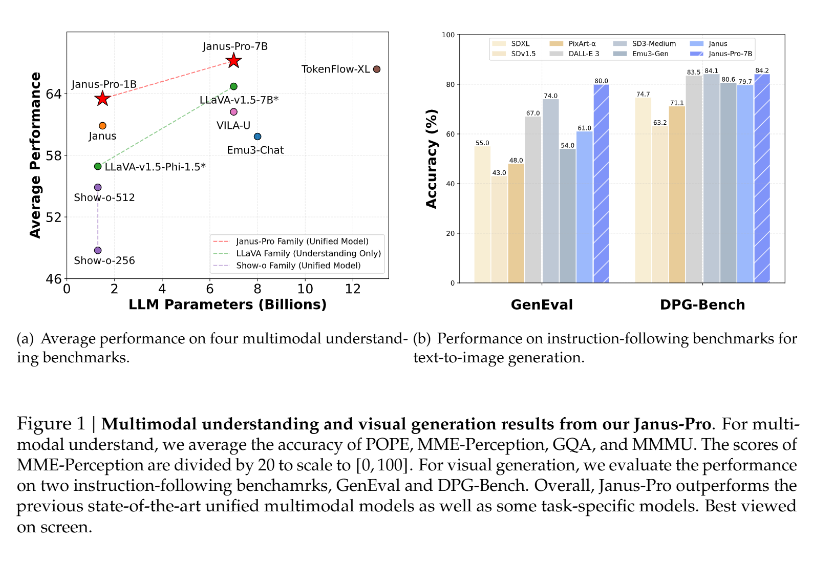
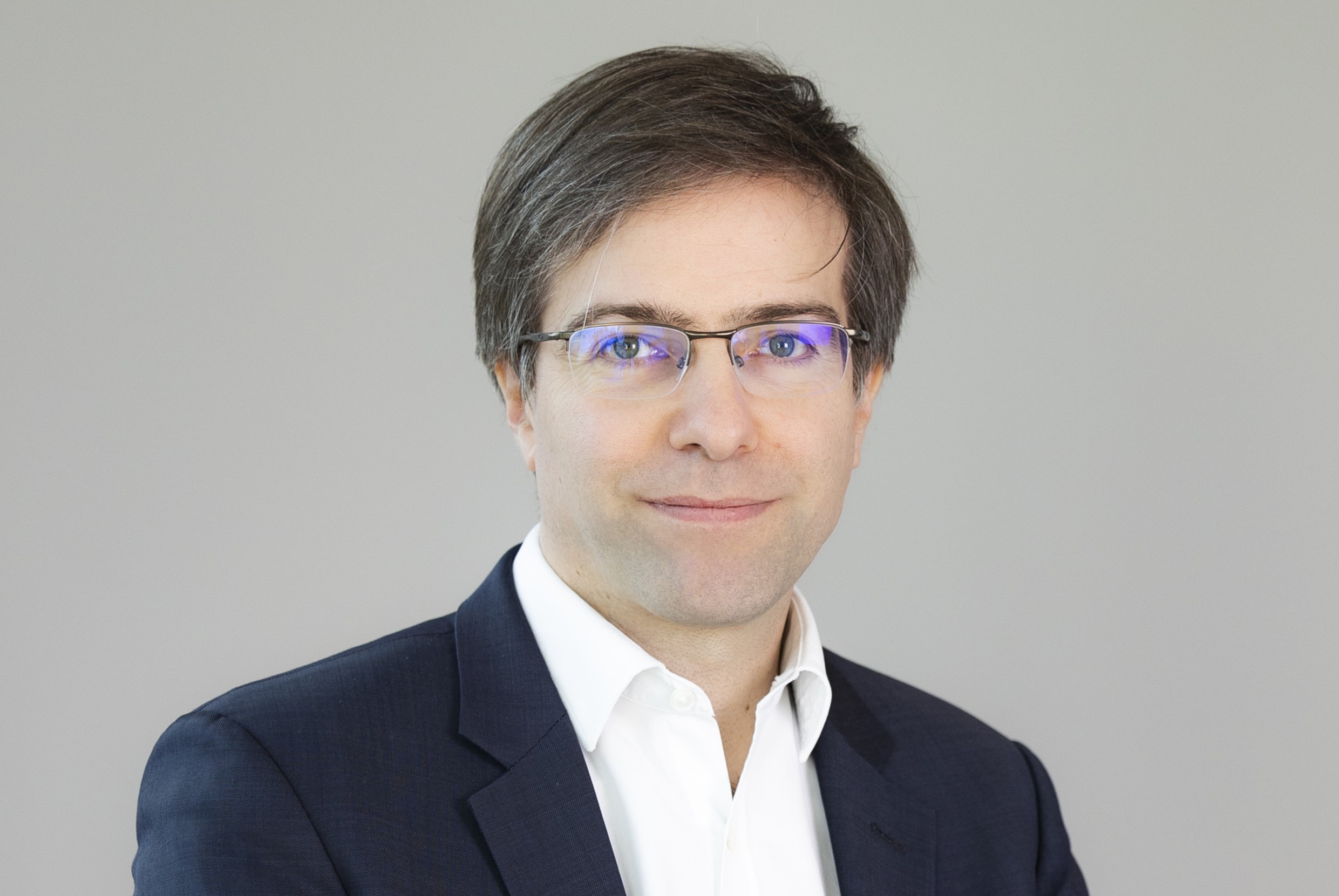
Emmanuel Hauptmann is CIO and Head of Systematic Equities at RAM AI. He co-founded the company in 2007 and has led the development of the firm’s systematic investment and AI platform since.